Real-time Exercise Analysis: Algorithmic vs Machine Learning Approaches in Motion Tracking
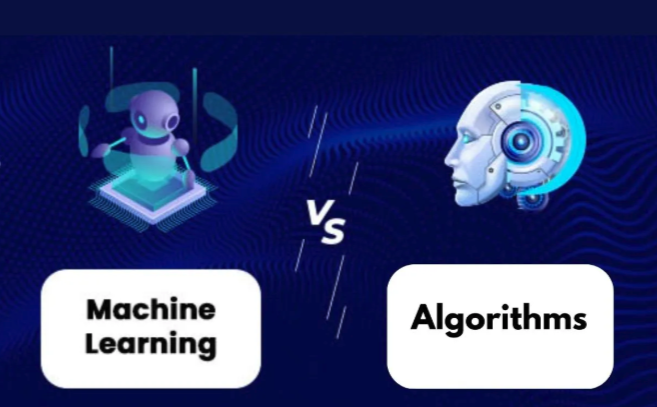
Real-time Exercise Analysis: Algorithmic vs Machine Learning Approaches in Motion Tracking
Introduction
In the rapidly evolving landscape of fitness technology, real-time motion analysis has become a cornerstone for providing interactive feedback during workouts. Two distinct approaches have emerged for implementing exercise recognition and form correction: algorithmic (rule-based) systems and machine learning classification models. Understanding the differences between these approaches is crucial for developers and fitness platforms looking to implement movement analysis features.
The Algorithmic Approach to Real -Time Motion Analysis
How Rule-Based Systems Work ?
Algorithmic exercise recognition uses predefined mathematical rules and biomechanical principles to analyze movements. This approach relies on:
- Geometric calculations between key body points
- Angular relationships between joints
- Velocity and acceleration patterns
- Specific movement threshold definitions
Advantages of Algorithmic Implementation
- Precise Standardization
- Exact movement criteria can be defined
- Consistent evaluation across all users
- Clear pass/fail conditions for form checks
- Real-Time Feedback Capabilities
- Immediate form correction
- Instant rep counting
- Low latency response
- Transparent Decision Making
- Clear understanding of why a movement was validated or rejected
- Easy to adjust and fine-tune parameters
- Predictable behavior
Implementation Timeline
- Initial Framework Development (3-5 days)
- Core geometric calculation engine
- Joint relationship analysis system
- Real-time validation pipeline
- Feedback generation system
- Per-Movement Implementation (~1 day per movement)
- Define movement-specific rules
- Set biomechanical thresholds
- Configure feedback triggers
- Testing and calibration
# Example of reusable core framework
class MovementAnalyzer:
def __init__(self):
self.joint_calculator = JointCalculator()
self.movement_validator = MovementValidator()
self.feedback_generator = FeedbackGenerator()
def analyze_movement(self, keypoints, movement_type):
angles = self.joint_calculator.compute_angles(keypoints)
validation = self.movement_validator.validate(
angles,
movement_type
)
return self.feedback_generator.generate_feedback(validation)
# Adding new movements becomes quick and simple
class SquatAnalyzer(MovementAnalyzer):
def __init__(self):
super().__init__()
self.movement_rules = {
'knee_angle': (80, 100),
'hip_depth': -0.3,
'back_angle': (70, 90)
}
Machine Learning Classification Approach
How ML Classification Works ?
Machine learning models learn movement patterns from video datasets, using:
- Training data of correct exercises
- Feature extraction from pose estimation
- Pattern recognition across multiple repetitions
- Probability-based classification
Advantages of ML Implementation
- Flexibility in Recognition
- Can handle variations in movement styles
- Adaptable to different body types
- Natural movement understanding
- Simple Initial Setup
- No need to manually define movement rules
- Can learn from example videos
- Quick to implement basic recognition
Implementation Timeline
- Initial Classifier Development (3-5 days)
- Model architecture design
- Training pipeline setup
- Feature extraction system
- Inference optimization
- Per-Movement Data Collection and Training (2-5 days per movement)
- Collect reference movement videos
- Record common error variations
- Label and annotate dataset
- Train and validate model
- Fine-tune for real-time performance
# Example of ML-based classification
class ExerciseClassifier:
def __init__(self, model_path):
self.model = load_trained_model(model_path)
def classify_movement(self, pose_sequence):
features = extract_features(pose_sequence)
prediction = self.model.predict(features)
return get_movement_class(prediction)
Challenges in Form Correction
- Data Collection Complexity
- Need examples of both correct and incorrect forms
- Multiple variations of each error type required
- Time-consuming dataset creation
- Feedback Precision
- Less specific error identification
- Probabilistic nature of corrections
- Harder to standardize feedback
Implementation Comparison Table
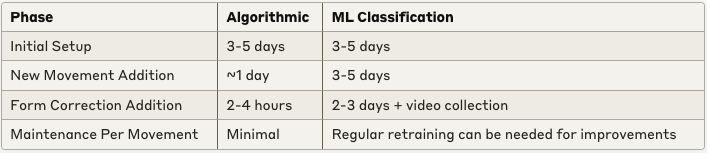
Real-World Implementation Example
At PoseTracker, we've implemented both approaches and found that algorithmic systems offer superior results for real-time exercise feedback. For example, our flexibility analysis tool uses precise geometric calculations to provide instant angle measurements and form corrections, achieving over 95% accuracy in movement validation.
The algorithmic approach particularly shines in applications requiring:
- Rapid deployment of new movements
- Standardized form correction
- Consistent real-time feedback
- Easy maintenance and updates
Conclusion
While both approaches have their merits, algorithmic implementations currently offer the most reliable and precise solution for real-time exercise feedback. ML classification excels at movement recognition but faces challenges in providing standardized form correction. The choice between the two approaches often depends on specific use cases:
- Choose algorithmic approach for:
- Precise form feedback requirements
- Quick implementation of new movements
- Standardized evaluation criteria
- Clear feedback requirements
- Consider ML classification for:
- Complex movement pattern recognition
- Handling significant movement variations
- Basic movement counting
- Less strict form requirements
Looking to implement motion analysis in your application? Explore PoseTracker's API solutions we provide the most advanced, accurate, and easy-to-integrate pose estimation technology for developers across industries. 🤓